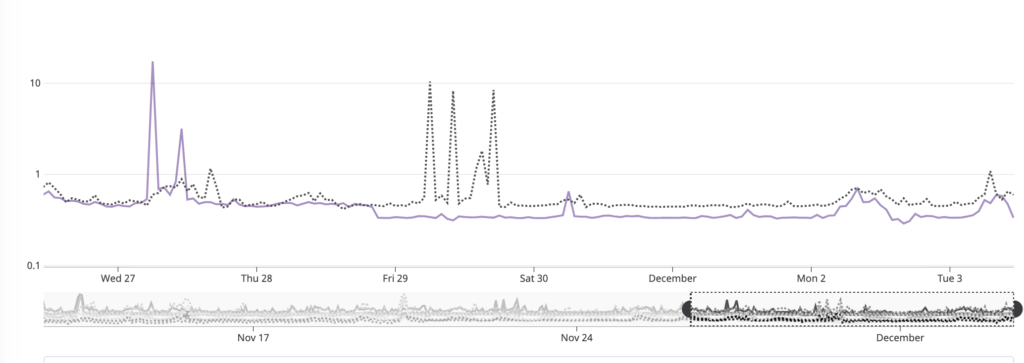
We use bcrypt to store passwords in Luma Health. In the NodeJS world there are two common libraries used to do this, bcryptjs, which is a pure JavaScript implementation and bcrypt, which is a wrapper on a C++ library.
We had originally used the pure JS version since it was helpful when we upgraded NodeJS versions that linked libraries didn’t have different binary versions, which caused long recompile and npm install times.
Our original implementation (mostly out of laziness) used the synchronous version of the JS library. Since bcrypt is a cost-of-compute type of algorithm, any time we came under high login login (e.g. the start of the day), we started to run CPU hot and then start to time connections as the cost of doing password hashes started to starve out other work happening on our REST servers.
We diagnosed this using Clinic and generating flamegraphs of the system under standard load patterns and it became clear that the bcrypt work was sucking up all the oxygen in the NodeJS process.

Our first fix was to just move to the async methods of the bcryptjs library, which would mean we wouldn’t block the main event loop doing bcrypt’s hashes. Unforutnatly, this didn’t lead to as much of a performance imprvoement as we were hoping to get becuase after digging in to the implementation of bcryptjs (<3 open source), it turns out the main difference between the async version and sync version is that the library would do one round of blowfish per callback. It was definitly an improvement but it still consumed a lot of time within the main NodeJS process.
We then looked at moving to the C++ based module and again it had two different functions, one sync and another async. The sync one would run the hashing functions in C++, which is faster, but even better, the async version would run the hashing function in an nan::AsyncWorker.
Result? About a 30% increase in response times through our front ends a lot smoother load management under high load. Moral of the story? Always use async even when it’s tempting / easy / lazy to use a sync version of a function in NodeJS. The graph from the very beginning shows the improvements right after we deployed the new code to production.